Grow Your Content Business with The Tilt
Join the community supporting content creators and entrepreneurs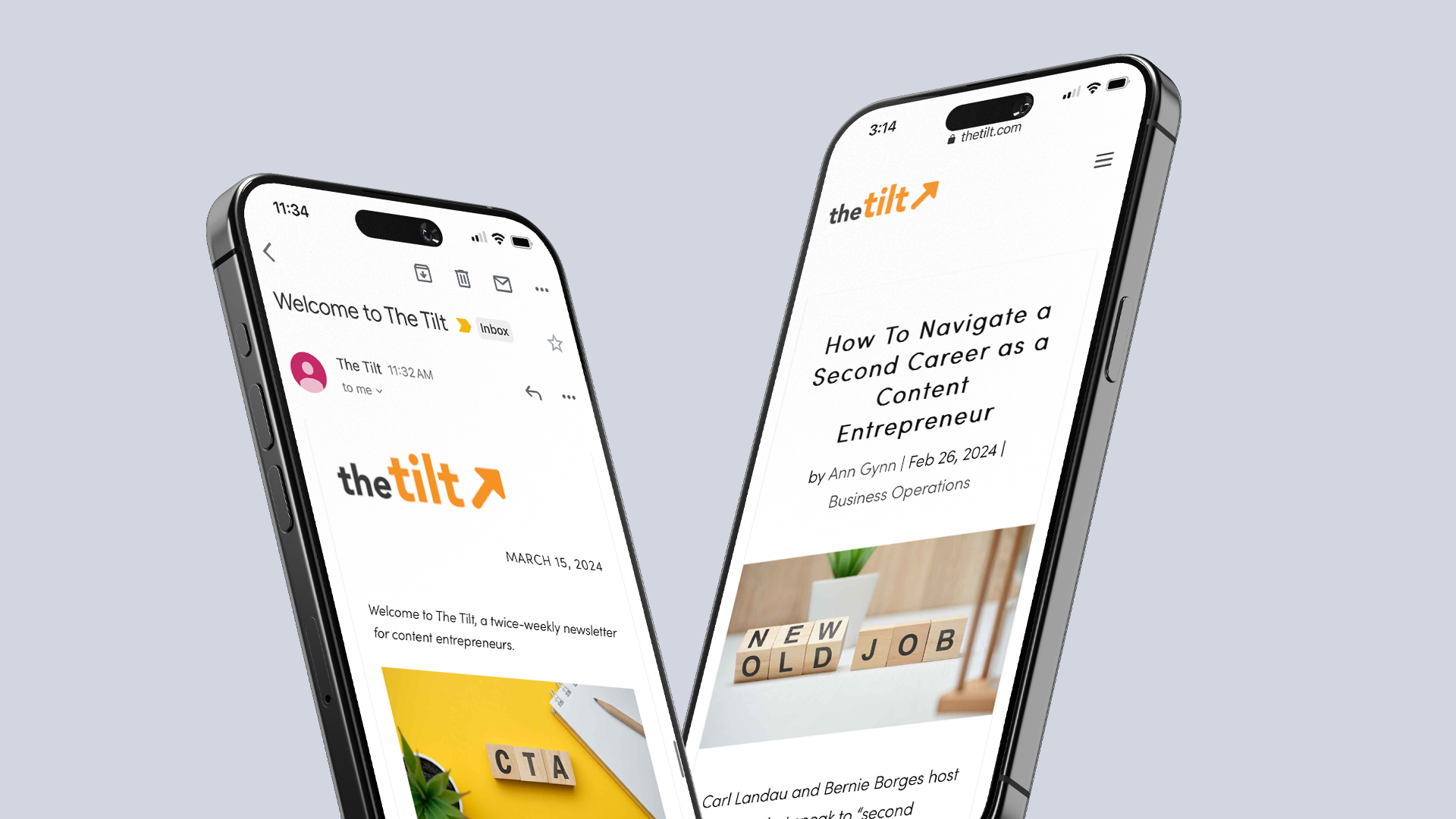
Join the Tilt Newsletter
We deliver valuable information for every content entrepreneur in our biweekly newsletter. Build a financially successful business alongside 30k other content creators and entrepreneurs who trust The Tilt newsletter!
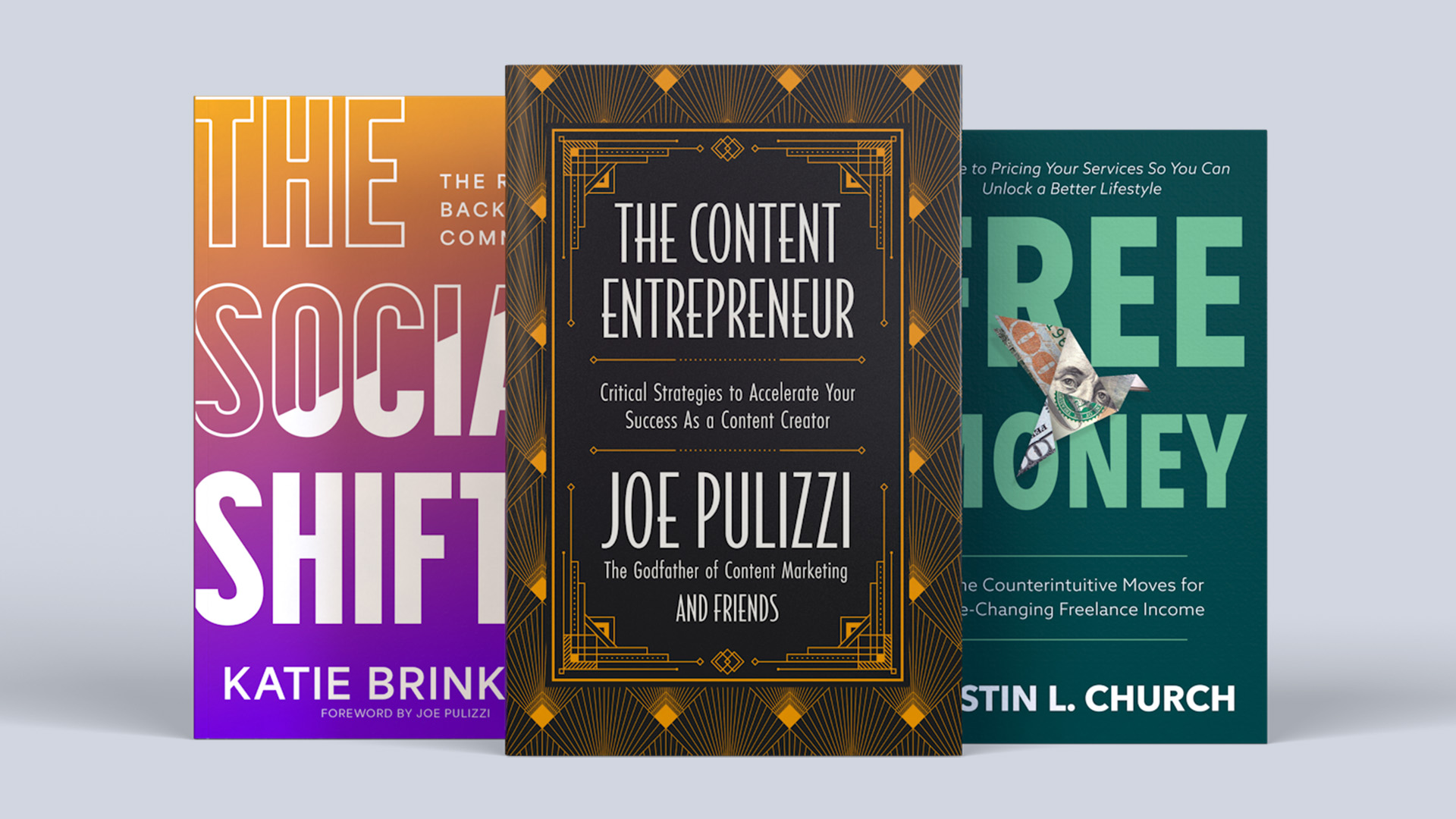
Publish Your Book with Tilt Publishing
Establish yourself as a true authority in your niche! Now is the time to add a book to your content business. Let us help you diversify your revenue streams, own your customer data, and grow your brand with our publishing services.